Preliminary Program
9-12 September 2025
9 September 2025 | 10 September 2025 | 11 September 2025 | 12 September 2025 | |
Workshop | Day 1 | Day 2 | Day 3 | |
9:00 | Introduction | Keynote: Maik Boltes |
Keynote: He Wang |
Keynote: Simo Hostikka |
Part I | Sessions: EXP-1, CM |
Sessions: EXP-3, ML-1 |
Sessions: EXP-5, MOD-5 |
|
12:00 | Lunch | Lunch | Lunch | Lunch |
Part II | Sessions: BEH-1, MOD-1 |
Sessions: BEH-2, MOD-3 |
Sessions: EXP-6, ML-2 |
|
15:00 | Break | Poster | Poster | Farewell drink |
Part III | Sessions: EXP-2, MOD-2 |
Sessions: EXP-4, MOD-4 |
||
17:00 | ||||
19:00 | Welcome drink | Social event | Dinner |
Oral contributions are assigned to sessions according to their main topics. You can find your preliminary time slot in the list below using your contribution's EasyChair ID.
Experiments and data
- EXP-1: 24, 68, 88, 99,
- EXP-2: 58, 84, 95,
- EXP-3: 62, 79, 82, 94,
- EXP-4: 23, 90, 60,
- EXP-5: 85, 89, 115, 21,
- EXP-6: 16, 34, 59, 66.
Modeling and simulation
- MOD-1: 78, 44, 67, 47,
- MOD-2: 101, 43, 119,
- MOD-3: 15, 40, 56, 113,
- MOD-4: 128, 26, 72,
- MOD-5: 65, 2, 19, 25.
Machine learning
- ML-1: 35, 106, 116, 122,
- ML-2: 36, 105, 108, 125.
Behavioral aspects and VR studies
- BEH-1: 57, 64, 97, 114,
- BEH-2: 110, 75, 81, 126.
Crowd management
- CM: 14, 92, 112, 3.
PhD Workshop
Topic: Data acquisition from pedestrian experiments
Content: Practical seminars in PC labs focusing on pedestrian experiments and pedestrian recognition and tracking.
Number of attendees is limited with priority of PhD students.
Keynote Speakers
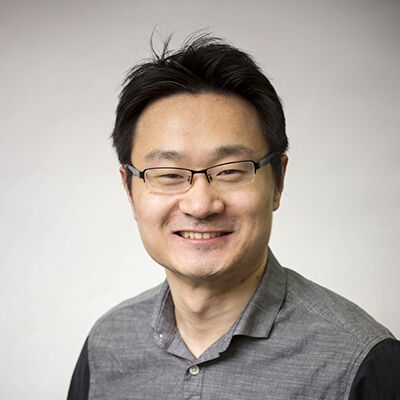
Understanding pedestrian and crowd movements is a crucial challenge spanning multiple disciplines, from mathematics, physics, and computer science to public safety, event planning, policymaking, and psychology. Decades of research have provided valuable insights and powerful analytical tools, and since 2016, deep learning has emerged as a transformative force in this field. In this talk, I will introduce our latest research on pedestrian dynamics within the deep learning landscape. Moving beyond traditional explicit models and black-box AI, a new trend has gained momentum since 2022—integrating physics-based models with deep neural networks. This hybrid approach enhances predictive accuracy, improves explainability, and strengthens generalization, paving the way for a deeper understanding of complex human movement patterns.
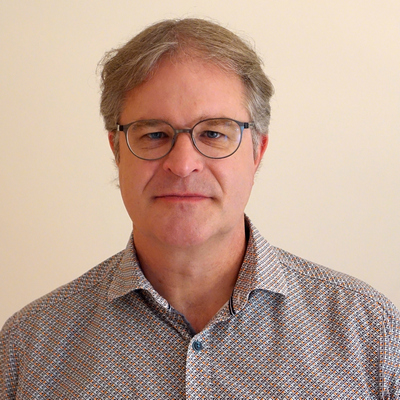
Empirical data is the basis for studying and thus understanding the dynamics inside crowds, which could increase safety and comfort for pedestrians as well as the performance of pedestrian facilities. The results enable the development of models reflecting the real dynamics. Controlled reproducible experiments allow the quantitative description of pedestrian dynamics by investigating influencing aspects and enable the analysis of selected parameters under well-defined constant conditions. Data of these experiments has to be collected by appropriately selected and utilized sensors.
In my presentation, I will address the implementation of laboratory experiments, with a particular focus on the collection of experimental data. I will discuss both the opportunities and limitations of various data collection techniques and methods, as well as their practical applications. The fusion of carefully calibrated and synchronized data enables the correlation of different influencing factors. Linking individual characteristics to specific subjects within a dataset makes it possible to analyze the impact of personal attributes on the specific dynamics. The use of standardized methods for data acquisition, measurement, and storing data significantly enhances the comparability of experimental results. Furthermore, the availability of open data and open-source software is essential for ensuring the reproducibility of findings and for facilitating the reuse of the often laboriously collected experimental datasets. These aspects will also be explored in my presentation.
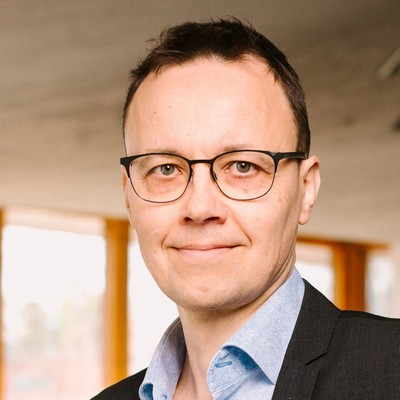
Fire and evacuation simulations are often conducted as part of a building’s design process to ensure that occupants can evacuate or be rescued in the event of a fire, or as part of a fire investigation to assess the conditions and timing of a past incident. Despite the clear interdependence between fire development and evacuation processes, these simulations are usually performed independently. This presentation will discuss the reasons for and extent to which these simulations should be coupled, the technical challenges involved, and development opportunities to support practitioners in analyzing scenarios that account for the interactions between fire and human behavior.
Key motivations for coupling fire and evacuation simulations include the need to evaluate potential toxic effects and reduced visibility due to smoke, which is often modeled through walking speed reduction. Wayfinding difficulties are typically addressed by applying scenario- and location-specific visibility thresholds. The adequacy of using visibility as a surrogate for irritation will be examined in light of literature data. Beyond wayfinding as a physical task, efforts have been made to predict evacuees’ decision-making processes; however, these methods have not yet matured into practical applications. Recently, increasing interest in wildfire evacuation has reignited this topic. In building fires, two-way coupling may also be necessary, as evacuee decisions could influence fire development.
Recent advancements in fire toxicity modeling have revealed that limitations in transferring toxicity data can lead to underestimated risks and non-conservative designs. This presentation proposes an approach to improve both the accuracy and computational efficiency of toxicity coupling by using effective surrogate species and optimizing the selection of transferred quantities. The potential role of Building Information Modeling (BIM) standardization in supporting these improvements will also be briefly discussed.